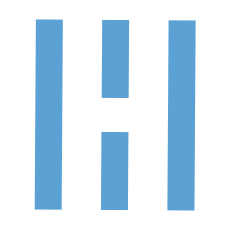
Big Data
Big data is a term for data sets that are so large or complex that traditional data processing applications are inadequate. We use deep learning to analyze multi-modal Big Data such as genomics or clinical imaging from patients to reveal patterns, trends, and associations with disease. We strive to ultimately translate Big Data from the clinics into useful medical intelligence to impact clinical decisions.
Precision Medicine
Precision medicine is an innovative approach to disease prevention and treatment that takes into account individual differences in people’s genes, environments, and lifestyles. We are building precision medicine tools for doctors and their patients to better understand the complex mechanisms underlying disease and to better predict which clinical or surgical interventions will be optimally effective.
Digital Health
Digital health is the convergence of the digital and genomic revolutions with health, healthcare, living, and society. Digital health is empowering people to better track, manage, and improve their own and their family's health, live better, more productive lives, and improve society. We develop medical intelligence to power digital health applications in the clinics to improve the precision of medicine for doctors and their patients.
Media
We hope you enjoy our productions.
Leveraging Voice Technologies on the Blockchain to Decentralize Clinical Trials
November 17th, 2022
Presentation at the Artificial Intelligence Blockchain (AIBC) Europe Conference on using AI to decentralize clinical trials in the Metaverse using voice micro services. Delivered at the Hilton in St. Julians, Malta.
How AI is changing the Face of Medicine: From Education to Practice
November 11th, 2022
Keynote at the annual Matheson Lecture of the Association of Academic Health Sciences Libraries in Nashville, TN.
Gamifying a Network of Patients Providers and Clinical Trials with AI
October 14th, 2022
Keynote at the Inaugural UCF WEALTH Symposium to showcase Wellness, Education, AI, & Lifestyle Technology in Health (UCFWEALTH.org) innovations being developed in our budding Lake Nona Medical City, Orlando, FL.
From Curation to Community-Driven AI
March 28th, 2022
Presented research funded by the National Institutes of Health at the inaugural Curation at Scale workshop as a forum hosted by National Library of Medicine for discussing challenges, opportunities and fostering collaboration in digital curation by and for the biomedical community.
Transforming Healthcare with
Community-Driven AI
October 14th, 2021
Keynote at Artificial Intelligence and Machine Learning: The Future is Now virtual conference bringing together key stakeholders to facilitate the adoption of artificial intelligence and machine learning technology.
Community-Driven AI at Meta-AI Summit by Deepak Chopra
July 21st, 2021
Moderated presentation about our vision for Justice, Equity, Diversity (JEDI) and Inclusion in AI at UCF College of Medicine for health science at the first inaugural virtual Meta-AI Summit hosted by the The Chopra Foundation by Deepak Chopra.
COVID-19 in the Caribbean
April 15, 2020
A vital discussion on COVID-19 among medical, scientific and public health experts from the Caribbean. Hear about the unique effects on our communities around the globe including thoughts on how we respond to such outbreaks in the future. Moderated by by Fzero Genomics, the Caribbean's premiere personal genomics company.
International Congress on Cancer Metastasis
October 26th, 2019
Presentation on translating routine clinical data into artificial intelligence at the 8th International Congress on Cancer Metastasis in San Francisco, California.
Heidelberg Laureate Forum
September 25th, 2018
Presentation on the importance of blockchain and sharing medical data in developing clinical grade artificial intelligence at the 6th annual Heidelberg Laureate Forum in Heidelberg, Germany.
Bloomberg The Year Ahead: 2018
November 8, 2017
Emma Chandra interviews Mike Cordano and Dexter Hadley at Bloomberg The Year Ahead on how Western Digital and UCSF will team up for breast cancer research. Delivered at Bloomberg headquarters in New York City.
From Bits to Bedside™
February 22, 2017.
Keynote at LabRoots Precision Medicine 2017 virtual event keynote presentation on translating big data into precision medicine and digital health at University of California, San Francisco.
Precision Screening and Diagnosis of Melanoma
November 7th, 2016
Three minute pitch at Science Match Future Medicine to use deep learning and smartphones for precision screening and diagnosis of skin cancer delivered in Berlin, Germany.
We Translate Big Data into Clinical Intelligence!
Practicing medicine involves first screening patients towards making definitive diagnoses and ultimately therapeutic interventions. We leverage big data and digital health to realize precision medicine for doctors and their patients. We are building the infrastructure to collect, connect, and apply vast amounts of scientific research data and information about our health. Our goal is to understand how individuals respond differently to interventions to help guide more precise and predictive medicine worldwide.
PRECISION SCREENING
We develop digital health applications to improve screening for disease. We use data from mobile health apps and medical imaging to monitor large numbers of patients in the clinics. Our goal is to save lives by improving digital surveillance that can alert physicians and their patients earlier about the risks of disease.
PRECISION DIAGNOSIS
We develop models of medical intelligence to improve diagnosis and management of disease. We leverage deep learning technologies to translate big data into diagnostic and prognostic intelligence. Our goal is to develop medical intelligence models that perform at the level of a specialist physician.
PRECISION THERAPY
We mine open data to understand defective patterns of genomics disease signatures. We integrate genomic signatures of drug response to select optimal agents that directly reverse disease genomics. Our goal is to develop more personalized therapeutic options to fight disease.
Our Research Impacts Patients.
We develop clinical intelligence models from big data that optimally predict disease and appropriate clinical management. We train our models on big data from a variety of sources including public molecular data repositories as well as protected private electronic health information such as clinical imaging . We leverage these models to power intelligent, self-learning predictive clinical applications designed to improve their performance with use over time. We also integrate our molecular models of disease with large databases to find novel biomarkers and novel therapeutics to manage disease.
Cancer screening with mobile apps
DIGITAL Risk assessment of melanoma
While melanoma is the number one killer of young adults with cancer, diagnosis is both subjective and imprecise. Overdiagnosis on clinical exam remains a problem with one melanoma on average found for every 30 suspicious skin lesions sent for biopsy. We are using serial imaging to develop a real-time non-invasive approach to improve precision in melanoma screening. We enroll patients in the dermatology clinics with apps that collect images of suspicious lesions on their skin. From this data we develop deep learning models of clinical intelligence to precisely screen for skin cancer from digital images. We build this clinical intelligence back into apps to provide accurate real-time surveillance of suspicious skin lesions for cancer. Our goal is to use digital health applications to save lives through more intelligent and precise screening for skin cancer.
Computational radiology for cancer
Precision mammography
Over 250,000 women will be diagnosed with breast cancer this year alone, but at least five million will be incorrectly screened by mammography and recalled for additional testing. To realize precision oncology for breast cancer screening, we train, optimize, and validate powerful deep learning algorithms on almost 5 million digital mammograms to more accurately detect clinically significant cancer, reduce anxiety from false positive screening, and decrease morbidity of unnecessary downstream procedures. Improved precision in screening for breast oncology may help tip the balance of routine screening towards greater benefit and less harm for millions of women.
Computational pathology for cancer
Drug repositioning for Medulloblastoma
Medulloblastoma is the most common malignant (cancerous) central nervous system tumor in children. It accounts for 15 to 20 percent of all pediatric brain tumors. Medulloblastomas occur most commonly in children between ages of 3 and 8 but can be seen in children and adults of any age. Molecular studies suggest medulloblastoma is not one disease but comprises a collection of at least four distinct molecular subgroups that differ in their clinical severity and response to chemotherapy. We are using our STARGEO genomics discovery platform to refine more precise genomic subtypes of melanoma, and we are looking for personalized therapeutics to make difficult to treat subtypes more amenable to standard chemotherapeutic regimens.
- Apps
We develop apps that engage patients, physicians, scientests and students to curate data for large scale machine learning in medicine.
THE COVID IMAGING PROJECT
is our IRB-approved study is to develop medical Artificial Intelligence (AI) to better understand COVID-19 using patients' chest imaging. In collaboration with clinical partners, we have built a secure platform that allows us to enroll patients who were previously hospitalized for COVID-19 to share their X-rays and clinical experiences. Our community of patients, clinicians, and scientists will make AI work for everyone.
Skin — Secure Login
Skin is a digital health imaging app for tracking of moles and skin lesions. The platform is targeted towards academic researchers, physicians and patients looking to integrate a robust sharing platform for the management and surveillance of dermatological conditions. This app gives medical institutions and physicians a secure option to create scalable studies with hundreds of patient participants. The tool is intended to help support the creation of an intelligent model for the accurate diagnosis of skin diseases.
Skin — Patient Profiles
Skin is a digital health imaging app for tracking of moles and skin lesions. The platform is targeted towards academic researchers, physicians and patients looking to integrate a robust sharing platform for the management and surveillance of dermatological conditions. This app gives medical institutions and physicians a secure option to create scalable studies with hundreds of patient participants. The tool is intended to help support the creation of an intelligent model for the accurate diagnosis of skin diseases.
Skin — Body Map
Skin is a digital health imaging app for tracking of moles and skin lesions. The platform is targeted towards academic researchers, physicians and patients looking to integrate a robust sharing platform for the management and surveillance of dermatological conditions. This app gives medical institutions and physicians a secure option to create scalable studies with hundreds of patient participants. The tool is intended to help support the creation of an intelligent model for the accurate diagnosis of skin diseases.
Skin — Anatomic Mapping
Skin is a digital health imaging app for tracking of moles and skin lesions. The platform is targeted towards academic researchers, physicians and patients looking to integrate a robust sharing platform for the management and surveillance of dermatological conditions. This app gives medical institutions and physicians a secure option to create scalable studies with hundreds of patient participants. The tool is intended to help support the creation of an intelligent model for the accurate diagnosis of skin diseases.
Skin — Image Capture
Skin is a digital health imaging app for tracking of moles and skin lesions. The platform is targeted towards academic researchers, physicians and patients looking to integrate a robust sharing platform for the management and surveillance of dermatological conditions. This app gives medical institutions and physicians a secure option to create scalable studies with hundreds of patient participants. The tool is intended to help support the creation of an intelligent model for the accurate diagnosis of skin diseases.
Skin — Image library
Skin is a digital health imaging app for tracking of moles and skin lesions. The platform is targeted towards academic researchers, physicians and patients looking to integrate a robust sharing platform for the management and surveillance of dermatological conditions. This app gives medical institutions and physicians a secure option to create scalable studies with hundreds of patient participants. The tool is intended to help support the creation of an intelligent model for the accurate diagnosis of skin diseases.
The Search Tag Analyze Resource (STARGEO)
is an open genomics discovery web platform to annotate digital samples housed within NCBI Gene Expression Omnibus (GEO) and to find robust genomic signatures of disease. We enlist the help of medical students without coding experience to generate genomic signatures of disease which we integrate with other large databases to discover novel biomarkers to diagnose or more optimal therapeutic interventions to treat disease. STARGEO is an NCI funded project through the NIH BD2K NIH initiative to characterize the functional genomics of disease with open data.
Executive Profiles
We've got the best people running the show!
Dexter Hadley,
MD, PhD
Principal Investigator
Dr. Hadley focused on genomics and computational biology during his medical education at Penn. His early work focused identifying genomic risk factors for pediatric neuropsychiatric disease such as autism and ADHD. After completing medical school and a surgical internship, he led a translational bioinformatics team at the Center For Applied Genomics at CHoP where his research contributed to an ongoing precision medicine clinical trial for ADHD. He trained in pathology at Stanford where he mined the open data for biomarkers of severe dengue hemorrhagic fever infection. His research now focuses on digital health to generate, annotate and ultimately reason over big data stores to improve the precision of medicine across the disease spectrum.
Ben Franc,
MD, MS
Clinical Collaborator
Dr. Franc, is a Professor of Clinical Radiology in the Nuclear Medicine subspecialty at UCSF, and he served as Chairman of Nuclear Medicine from 2009-2014 and concomitantly served in multiple leadership positions in professional societies and health policy entities. Dr. Franc established a research program at UCSF focusing on the development of new molecular imaging techniques including novel small molecule exploration and development techniques. He has developed programs for the early adoption and implementation of nuclear-based imaging and therapeutic technologies including the continued development of technologies for molecular imaging, His research leverages UCSF's expansive EMRs and imaging repository to explore the implications of molecular imaging in healthcare.
John Shepherd,
PhD
Radiology Collaborator
Dr. Shepherd is a Professor in the Department of Radiology and the Director of the Breast and Bone Density Imaging Group at the University of California, San Francisco. He is an expert in quantitative breast imaging as well as musculoskeletal imaging using x-ray absorptiometry techniques. Dr. Shepherd’s research interests involve quantitative imaging methods for tissue composition using x-rays. His mammographic methods are used extensively for research around the world and in the San Francisco Mammography Registry Program. Dr. Shepherd’s efforts are focused on the technical imaging aspects of the measurements of the body composition and the translation of these measurements into different fields of study.
Faiq Shaikh,
MD
Radiomics Consultant
Dr. Shaikh is a Molecular imaging physician, Informatician and a Radiomics/ML consultant. He trained in Imaging Informatics and Applied QIA/Radiomics at UPMC and currently does translational research in Immuno-oncology and Precision medicine at UCSF, Mayo and Stanford. Dr. Shaikh’s contribution to our lab includes expertise in cloud-based data sharing, informatics analytics, medical-IT liaison and Imaging genomics.
Trainee Profiles
We've got a top notch team behind the production!
Hari Trivedi, MD
Post-doctoral clinical fellow
Dr. Trivedi is currently a clinical fellow at UCSF, trained in radiology. Dr. Trivedi's expertise is in applying artificial intelligence to radiology through deep learning techniques for image analysis, language processing, and decision support. His current projects include automated MRI protocoling, automated cancer detection in mammography, and classification of free text clinical reports.
Maryam Panahiazar, PhD
Postdoctoral Fellow
Dr. Panahiazar’s is an expert in natural language processing (NLP). Her background is in text mining approaches to extract, predict, and share metadata. She has earned two PhDs, one in computer science and the other in bioinformatics, and she was a former postdoc at the Center for Expanded Data Annotation and Retrieval (CEDAR) at Stanford as well as the Mayo Clinic. She implemented the ContextAware recommendation engine for meta data submission in CEDAR for GEO, and is extending it to BioSpecimens. Her NLP work today focuses on deep learning clinical contexts from EMR data and medical reports.
Dmytro Lituiev, PhD
Postdoctoral Fellow
Dr. Lituiev is an expert in deep learning. He is the only certified NVIDIA Deep Learning Ambassador at UCSF, and he also teaches advanced data science courses at UC Berkeley. He hosts the weekly SF Deep Learning Study Group at UCSF where a community of deep learning practitioners across SF Bay Area, from both academia and industry, meet to review state-of-the-art DL approaches and tackle novel projects. He has earned a PhD in computational biology, and his passion is to develop machine learning and visualization tools, for Life Sciences, and other applications. Dr, Lituiev strives to turn demands of experimentalists into automatized solutions by applying statistical, image and signal processing and statistical tools.
Andrew Wong
Pre-doctoral Medical student
Andrew is a UCSF medical student with a background in computer science berkeley. He is an avid computer programmer and software engineer and his interested lie in deep learning delirium screening from routine medical care and building optimal models for medical image recognition from siloed data. Andre won the prestigious Short Term Deans Prize in 2017 for his work predicting a patients risk for delirium from electronic medical records.
April Liang
Pre-doctoral Medical student
April is a UCSF medical student who majored in Computer Science at Princeton University. Her goal is to apply computational tools to improve health care quality, accessibility, and efficiency. Her research interests include automating the extraction of key information from narrative clinical documents, using computer vision to improve breast cancer screening, and applying machine learning to the prediction of preeclampsia risk.
Abhishek Bhattacharya
UNDERGRADUATE student
Abhishek is an undergraduate honors student at UCSB majoring in Computer Science and Biology. He is a member of the prestigious College of Creative Studies at his campus which recognizes a select group of students for their excellence in academics and research. In the last few years, he has worked with leading scientists at Stanford, UCSF and UCSB to uncover the potential of big data in computational biology. He is an avid computer programmer and software engineer, and his interests lie in deep learning precision interpretation of medical imaging for the clinics. He is involved with a number of ongoing projects in skin and breast cancer quantitative image analysis.
Henry Zhu
UNDERGRADUATE student
Henry is an undergraduate student at UC Berkeley majoring in Electrical Engineering and Computer Science (EECS) and Mechanical Engineering. He joined the lab through a collaboration with the Berkeley Institute of Data Science and is currently working on image recognition and classification of breast screenings, specifically on extracting information from pathology reports to efficiently generate large datasets.
Alumni Profiles
Our alumni go on to do great things!
Jordan Spatz, PhD
Post-doctoral medical Student
Dr. Spatz is a medical student at UCSF with a focus on practicing medicine and biomedical scientific inquiry in space. Dr. Spatz trained at MIT in bioastronautics and worked for Northrop Grumman to design aeronautical transportation. Dr. Spatz is mining the open data with STARGEO to find novel approaches to treat refractory breast cancer.
James Pan
Pre-doctoral medical Student
James is a neurosurgery bound medical student at Stanford University. He studied design at Carnigie Mellon University and performed research in neurosurgery. James' interests lie in brain cancer and he is actively using STARGEO to mine the open data to reposition drugs for medulloblastoma.
We need superstars to help us do awesome work!
We are looking for passionate and motivated candidates who think Big Data can change everything about the delivery of medicine and healthcare. We work closely with clinicians to test hypotheses that we formulate in the laboratory. To apply for any of these positions, please send your CV, a brief statement of research interests, and contact information for three references in one PDF document to dexter.hadley@ucsf.edu.
Postdoctoral Fellows
Our laboratory is particularly geared to recruiting combined MD, PhD candidates who seek to make a translational impact in the clinic. We are ideally looking for candidates who have already earned a MD and/or PhD degree with a strong background in bioinformatics, computational biology, biostatistics, and genomics. A great publication record is not required, but a strong desire to publish is. Strong problem-solving skills, creative thinking, and the ability to build new software tools as needed are required. Applicants must possess good communication skills and be fluent in both spoken and written English. A background in molecular biology or medicine or pharmacology will be a strong plus. Prior experience with genetic, genomic, clinical imaging, biomarker discovery, drug repositioning is recommended but not mandatory. Familiarity with machine learning, text-mining, knowledge representation, and high performance parallel computing platforms and building web and mobile applications is a definite plus.
Medical Students
We love med students and offer ample opportunities for them to get involved with our translational research. No coding required! We have medical students using STARGEO to estimate robust signatures of disease genomics from which we propose novel biomarkers or drug repositioning strategies for disease. We have medical students in the clinics that collect patient data with apps that feed our self-learning models of clinical intelligence. As a medical student, we will help to devise a computational project that aligns with you clinical interests. Your expected effort will be variable and contingent on your clinical commitments. We will provide you with many opportunities to publish your clinical and / or basic science research in peer-reviewed journals, and we encourage medical students to present their work at medical/scientefic conferences around the world. Finally. we offer you ample opportunities for mentoring and professional networking that may illustrate alternative careers in medicine for you to pursue that drive innovation in health care and embrace translational research.
Predoctoral Students
We are looking for graduate rotation students to commit 50% of their time working on our research projects. Rotating students will be provided space in our shared office-space in Mission Hall. You will meet with the PI on a bi-weekly basis. You will interact with our Post-doctoral Fellows and graduate students. You are expected to attend our weekly laboratory meetings where your will present your rotation work. You are strongly encouraged to submit a paper on your work, even though it is just a rotation projectWe will expose you to a variety of computational frameworks and techniques that include:
- Python for open-source machine learning
- R for open-source statistical software
- Postgres for open-source relational database management..
Contact Us
Don't be afraid to reach out. You + us = awesome.
UCF College of Medicine 406D
6850 Lake Nona Blvd
Orlando, Fl 32827Lab Meetings every Wednesday, 12 NoonScheduling
Lets get in touch...
© 2016-2018